Mapping machine intelligence 
We develop a comprehensive model of AI tasks and datasets, the Intelligence Task Ontology and Knowledge Graph (ITO).
Accelerating research
Even though progress in AI has been impressive, it remains largely unclear how AI can be created and utilized to support scientific knowledge creation, discourse and decision making most effectively.
We analyze how human and machine intelligence may interact to accelerate scientific and societal progress.
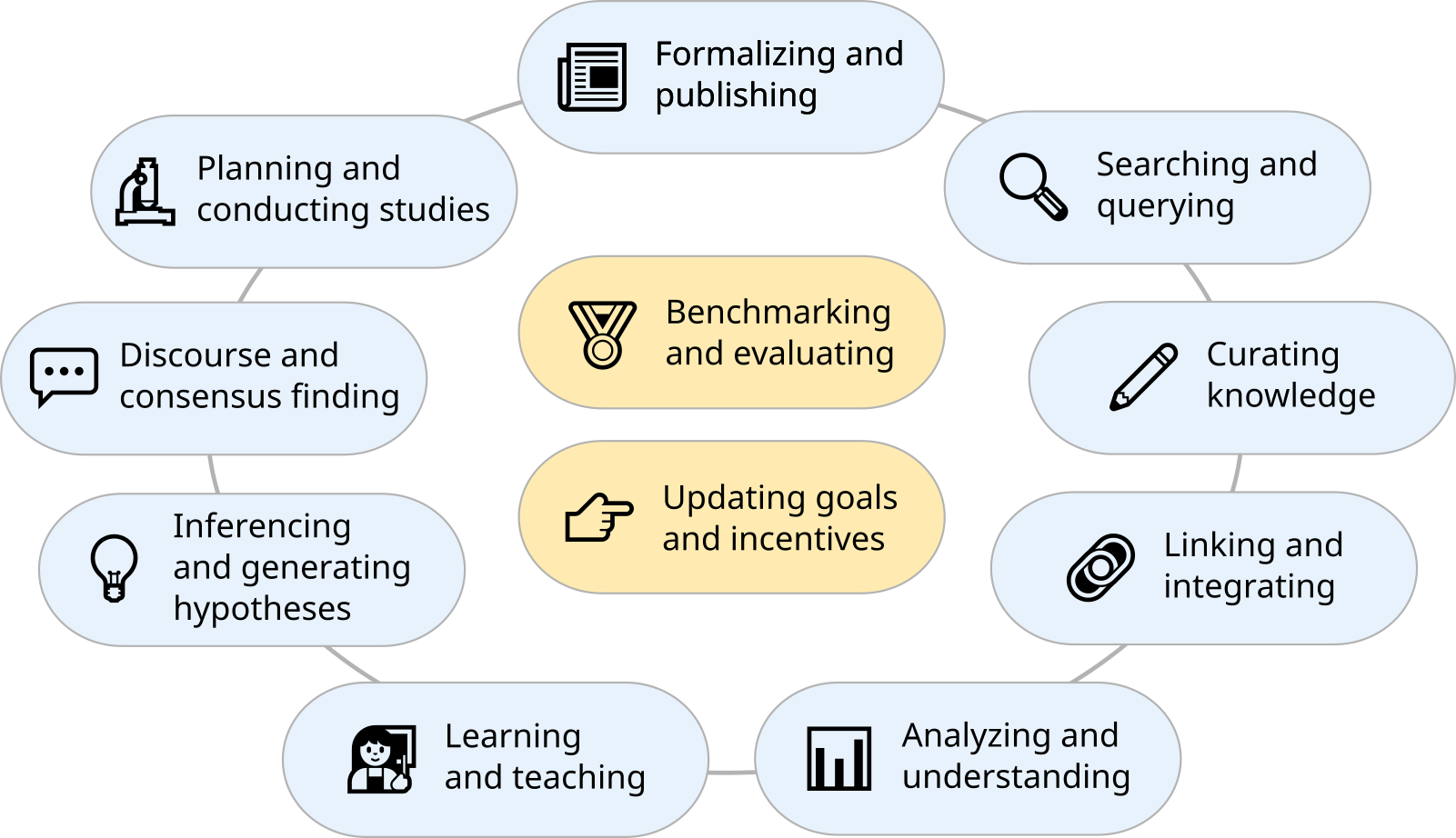
Mitigating risk 
Progress in the creation of transformative AI introduces various risks, such as the involuntary introduction of various types of biases by AI algorithms, voluntary manipulation and dominance of discourse, or AI-assisted gain of problematic technological capabilities.
To ensure that the overall impact of AI will be beneficial, we identify such risks and develop mitigation strategies.
Community 
We are creating an international community developing the theory and practice of scientific progress augmented through AI.
Publications & talks 
Barbosa-Silva, Ott, Blagec, Brauner, Samwald. “Mapping Global Dynamics of Benchmark Creation and Saturation in Artificial Intelligence”. arXiv (2022)
Blagec, Kraiger, Frühwirt, Samwald. “Benchmark Datasets Driving Artificial Intelligence Development Fail to Capture the Needs of Medical Professionals”. arXiv (2022)
Ott, Barbosa-Silva, Samwald. “LinkExplorer: Predicting, Explaining and Exploring Links in Large Biomedical Knowledge Graphs”. Bioinformatics (2022)
Blagec, Barbosa-Silva, Ott, Samwald. “A curated, ontology-based, large-scale knowledge graph of artificial intelligence tasks and benchmarks”“. arXiv (2021)
Moradi, Blagec, Haberl, Samwald. “GPT-3 Models are Poor Few-Shot Learners in the Biomedical Domain”. arXiv (2021)
Moradi, Samwald. “Evaluating the Robustness of Neural Language Models to Input Perturbations”. EMNLP conference (2021)
Moradia, Samwald. “Explaining Black-Box Models for Biomedical Text Classification”. IEEE Journal of Biomedical and Health Informatics (2021)
Ott, Meilicke, Samwald. “SAFRAN: An Interpretable, Rule-Based Link Prediction Method Outperforming Embedding Models”. 3rd Conference on Automated Knowledge Base Construction / AKBC (2021)
Samwald. “Aligning Human and Machine Intelligence”, Keynote talk @ Bio-Ontologies workshop, ISMB conference, (2021)
Blagec, Dorffner, Moradi, Samwald. “A critical analysis of metrics used for measuring progress in artificial intelligence”. arXiv (2020)
Breit, Ott, Agibetov, Samwald. “OpenBioLink: A benchmarking framework for large-scale biomedical link prediction”. Bioinformatics (2020)